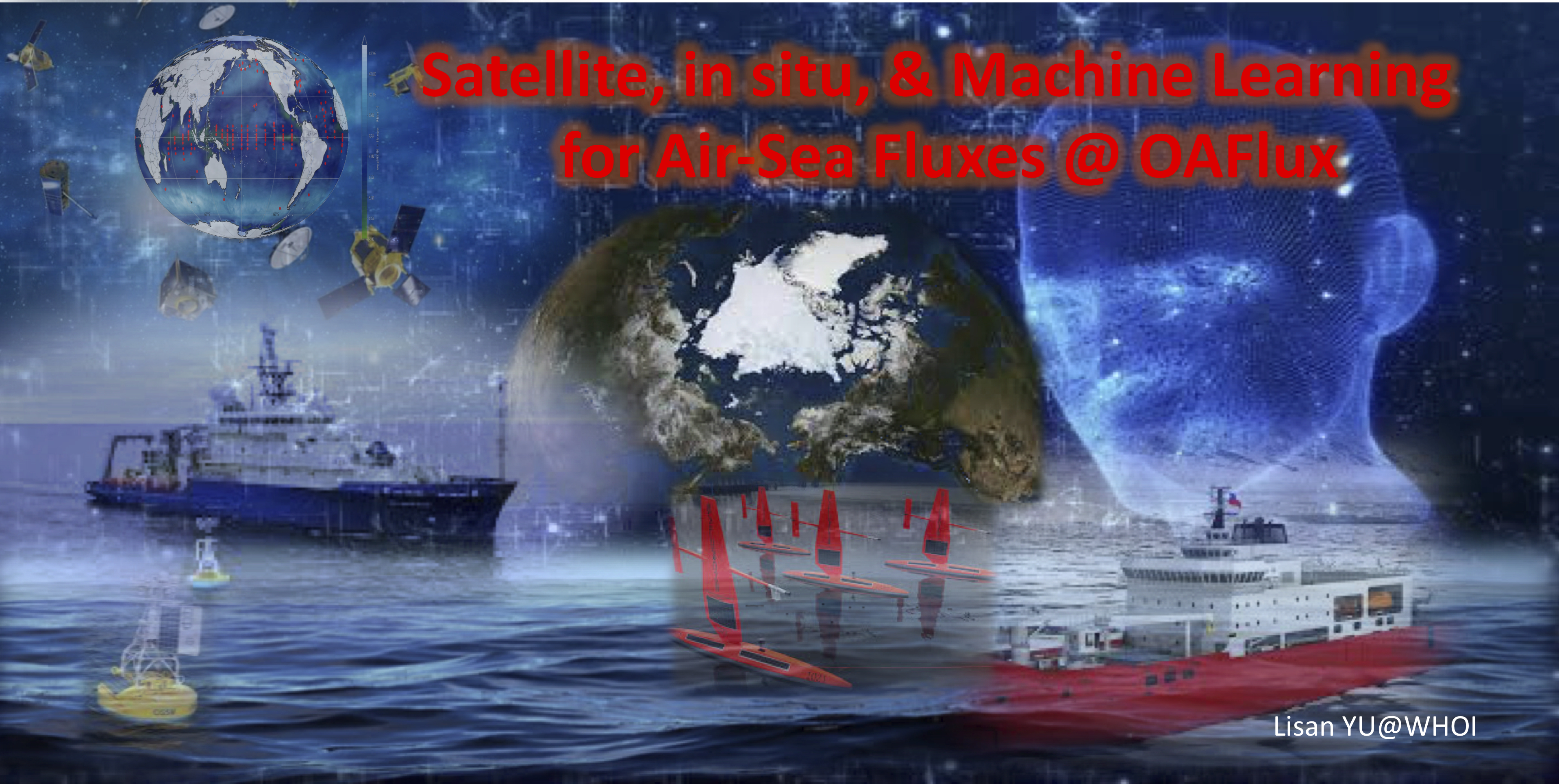
The air–sea turbulent heat fluxes are fundamental processes for keeping the global climate system in balance with the incoming insolation at Earth’s surface, and they are also a primary conduit for coupling and feedback between the ocean and atmosphere on a broad range of scales, from synoptic weather events to regional and global circulation systems. However, these fluxes are notoriously difficult to observe by either in situ platforms or remote sensing technology. On a global scale, fluxes are computed from bulk parameterizations using near-surface meteorological variables (including wind speed (W), SST (Ts), near-surface air temperature (Ta) and humidity (qa)) as input. Satellite provides direct retrievals for W and Ts, but not for Ta and qa. The latter two variables are commonly extracted from satellite-measured column-integrated water vapor and/or atmospheric sounding units. This approach has two major caveats. One is the lack of a complete theory regarding the relationship between the near-surface thermodynamical variables and the total precipitable water. The other is that the retrieval algorithms are typically based on empirical relations and have many limitations. As a results, satellite-derived Ta and qa have large uncertainties.
Machine learning (ML) provides a highly interesting alternative to the traditional methods. ML is a form of Artificial Intelligence (AI) that enables a system to learn from data rather than pre-programming rules. The ML techniques have proved immensely useful for applications for which there are no clear theoretical predictions yet which are of significant. They can automatically analyze and learn a highly accurate predictive or classifier model by leveraging training datasets, learning algorithms, and optimization techniques. At OAFlux, we are building ML models for automated learning and discovery of the relationships and patterns of air-sea fluxes in satellite observations. Our current emphasis is on the Arctic fluxes supported by NOAA Climate Observations and Monitoring (COM) program.
